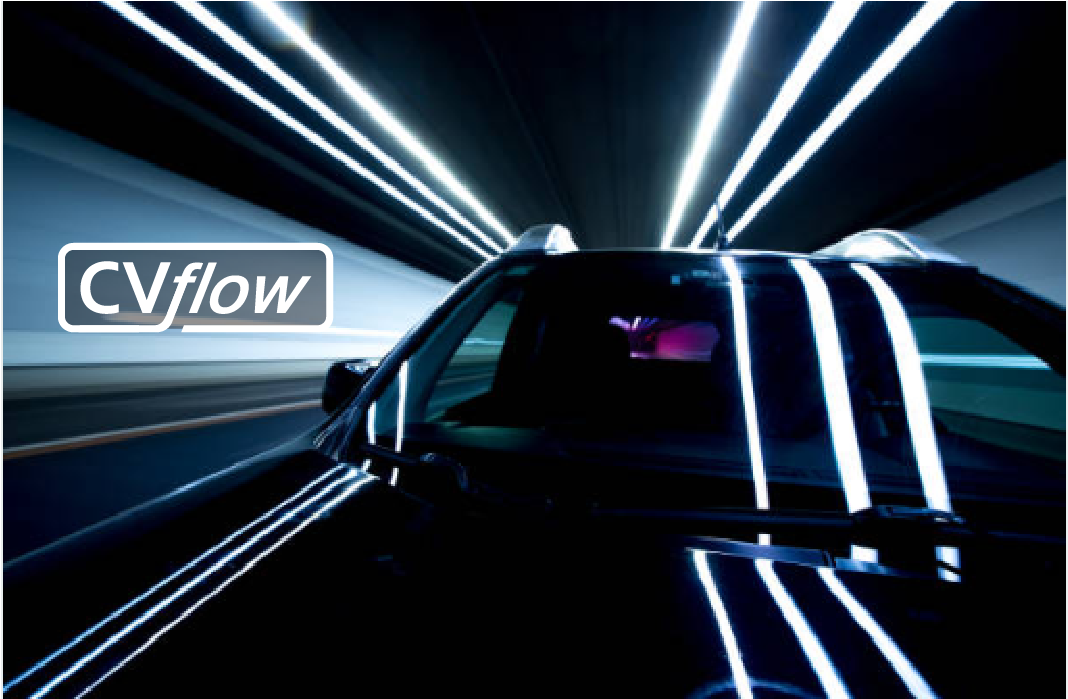
Modern automotive sensing applications require tailored AI accelerators.
When it comes to artificial intelligence applications in automotive, especially those involving data-intensive or sensor-driven operations, dedicated AI hardware has become essential.
These AI accelerators significantly outperform their general-purpose counterparts—GPUs or FPGAs—delivering powerful computing performance at significantly reduced power consumption.
“The entrance of Artificial Intelligence, bringing with it dedicated hardware called accelerators, enables increased understanding of the environment around the car and can therefore allow more and more autonomy as the software and the hardware are improving.“
Yole Développement (Yole), “Artificial Intelligence Computing for Automotive” report, May 2020
Ambarella’s AI accelerator, known as CVflow®, was specifically designed for high-performance computer vision, neural network processing, and machine learning in automotive applications. The result? CVflow-based solutions are capable of running multiple neural networks simultaneously, with each network trained to detect a different class of objects or perform complex segmentation of image content in a variety of traffic scenarios—all without consuming excess power or requiring unrealistic, non-production-ready form factors.
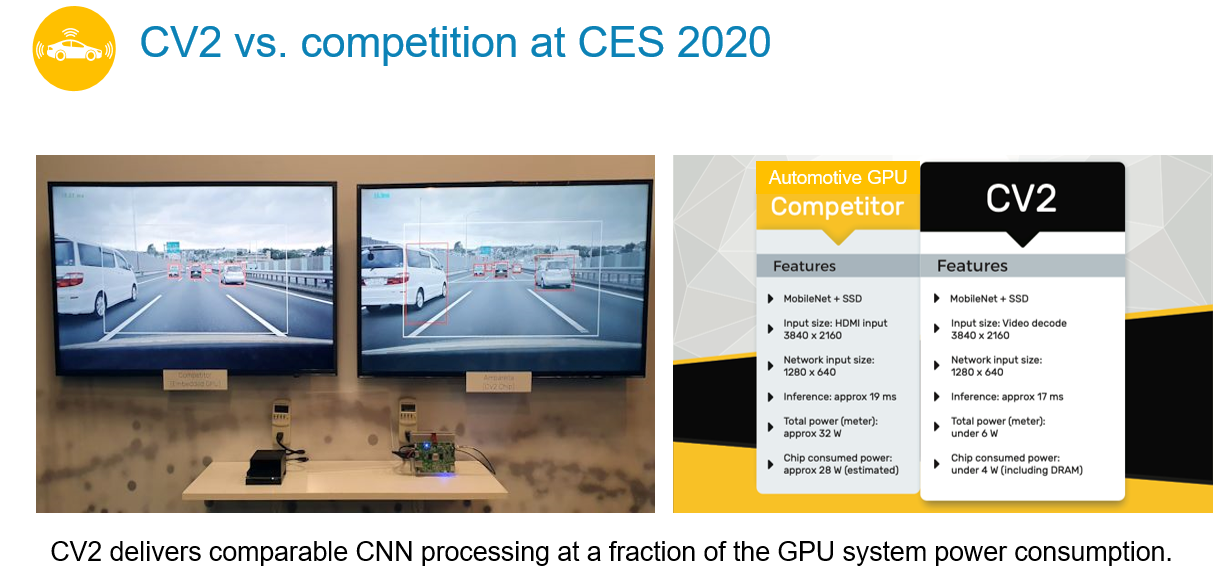
Our CVflow-based SoCs also feature the Arm Cortex-A53 processor, allowing the parallel execution of intelligent tasks such as object tracking or decision making, alongside traditional computing tasks.
A brief CVflow case study: the front ADAS camera.
To help illustrate CVflow’s benefits, let’s look at front-facing ADAS cameras, rapidly becoming a fixture in modern vehicles. As a standalone device, this camera must deliver fast and complex computer vision processing on the device itself within a strict power budget—typically under 5 watts for the entire system. Because of its location on the vehicle (toward the top of the windshield), a front ADAS camera is required to be relatively compact and sleek, occupying a minimum amount of space without sacrificing performance.
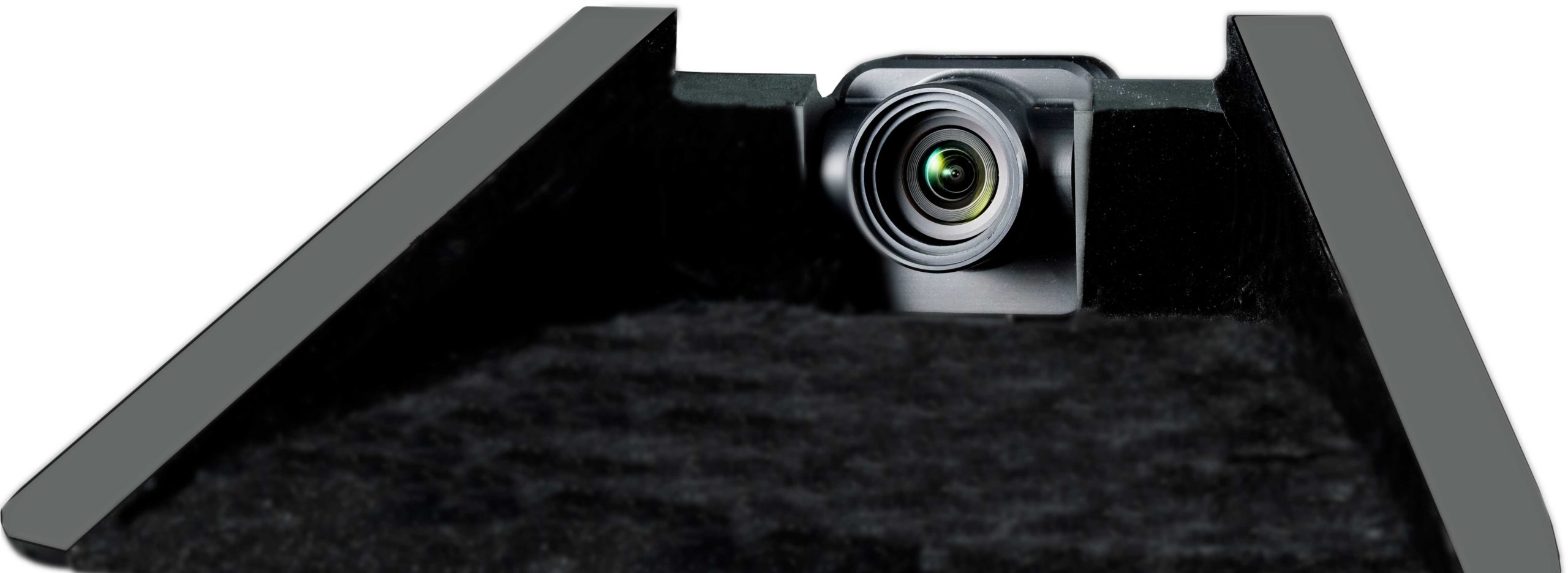
The device must detect a massive number of objects, apply complex behavior models to estimate and predict their movements in a dynamic scene, deliver timely warnings to the driver with high accuracy, and even trigger the execution of critical interventions such as emergency braking.
Front ADAS cameras currently in mass production often feature single cameras with resolutions between 1 and 2 megapixels, a relatively modest technical requirement. However, next-generation front ADAS cameras are expected to achieve 8-megapixel resolutions for increased sensing coverage at long distances, as well as wider-angle views at shorter distances. With CVflow, our computer vision SoCs are able to meet this daunting challenge—enabling low-power, high-performance, automotive AI at the edge using high-resolution imagery.
“We chose Ambarella’s CVflow SoCs due to their ability to deliver extremely high computer vision processing performance with very low power consumption.”
HELLA Aglaia
What are the key features of CVflow?
- Flexible neural network accelerator designed to efficiently map CNNs/DNNs trained with industry-standard tools such as TensorFlow, PyTorch, Caffe, or ONNX
- Scalability across different price/performance points
- Flexibility to address a variety of neural network architectures
- Low DRAM bandwidth, low latency, and low power consumption
- Robust set of software tools
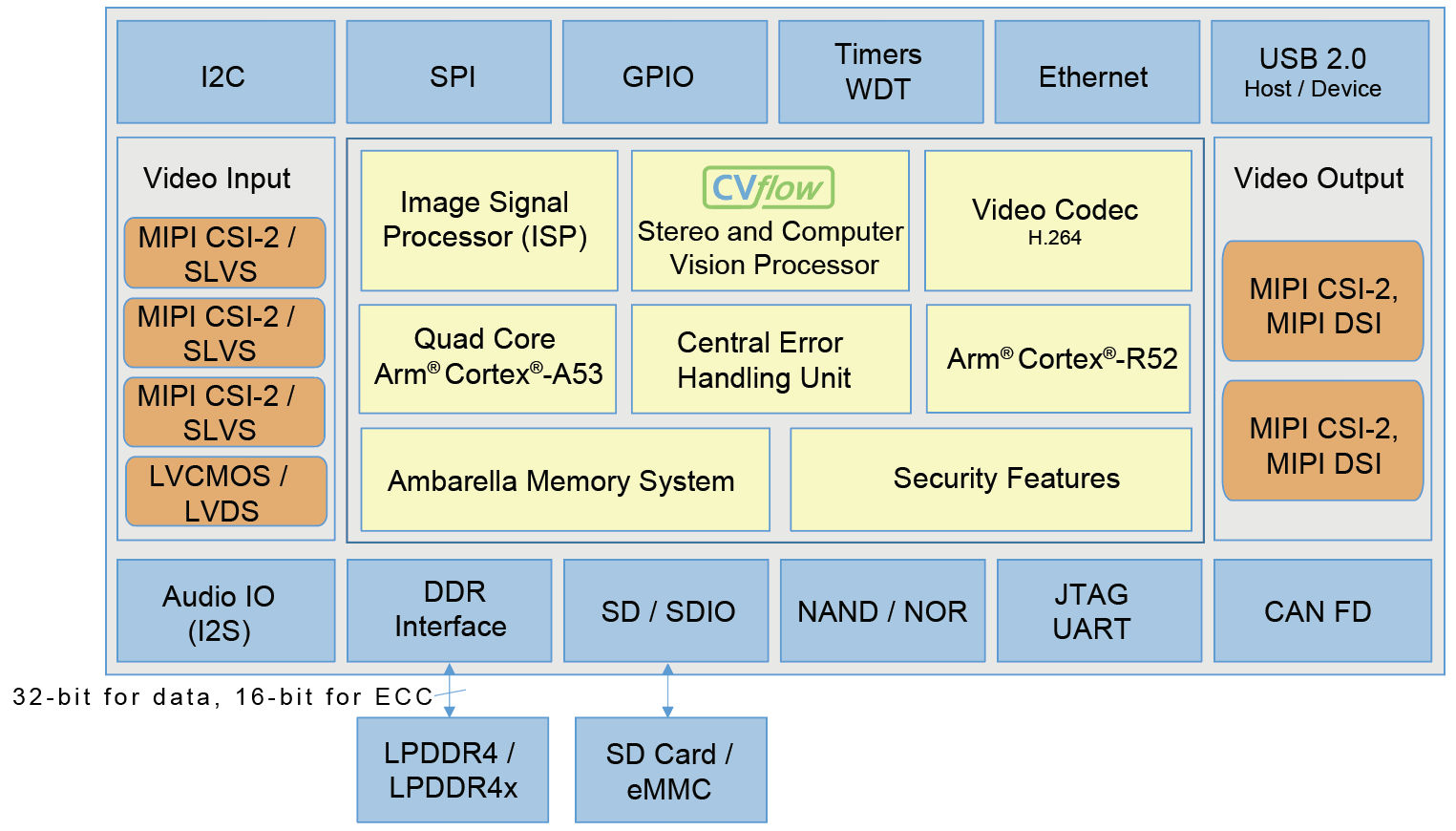
Click here for more information regarding our CVflow architecture.
For additional information, please contact us.